Long Beach, California—It is the heart of the CVPR 2019 Conference, bringing record attendance from 68 countries and a record number of paper submissions: The technical program.
With 1,294 research papers accepted from a record pool of 5,160 submissions this year, the authors’ technical presentations offered seemingly endless opportunities to listen and learn.
Only 288 papers were admitted into the so-called “oral sessions,” or presentations. Conference organizers reviewed and rated all papers, and the highest-rated papers were granted an oral presentation.
All 1,294 papers and their authors, however, were allowed to present their work in the conference’s poster sessions, so if a paper wasn’t granted an oral presentation, the authors could present their work on an eight-foot-by-four-foot poster on the exhibition floor or elsewhere in the Long Beach Convention & Entertainment Center.
Indeed, the volume of papers prompted organizers to limit each oral presentation to five minutes, in contrast to a typical limit of 15 minutes or so. Presentations were grouped in threes, for a total of 15 minutes, plus three minutes for questions about any of the three papers as a group. Then it was on to the next group of three.
The fast-paced presentations lasted over three days, with overflow rooms equipped with a big-screen feed from the packed ballrooms where the presentations occurred.
Here is a peek at just a handful of the paper presentations during the weeklong conference. The 2019 IEEE/CVF Conference on Computer Vision and Pattern Recognition (CVPR) is the premier event for technology professionals leading computer vision, machine learning, and artificial intelligence — the sectors behind such innovations as self-driving cars, facial recognition, and social media apps.
Paper Title: “Meta-Learning with Differentiable Convex Optimization”
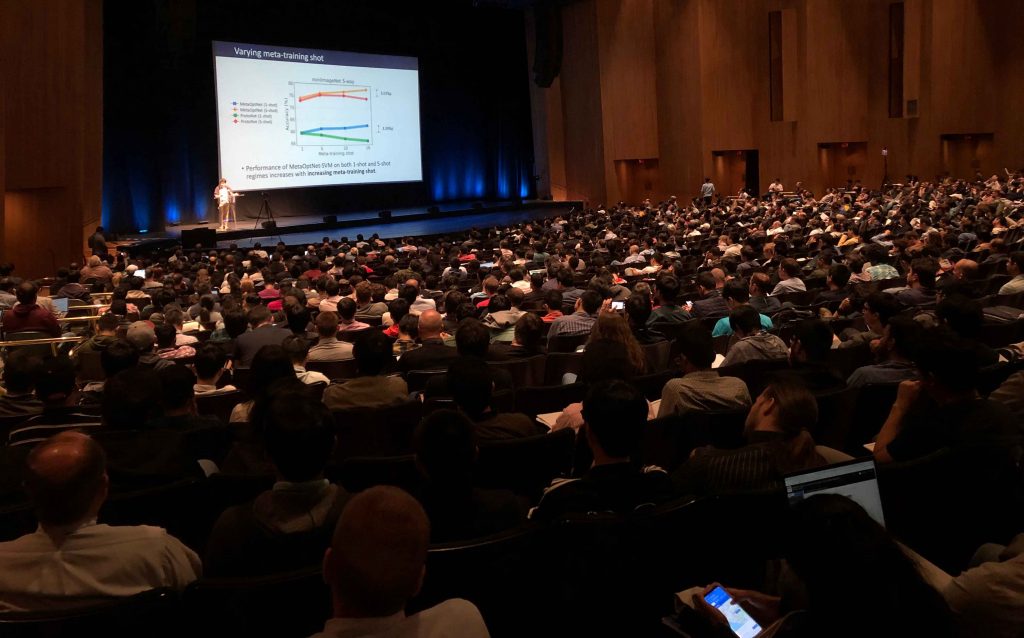
Of the three venues for the oral sessions, the 1,758-seat Terrace Theater was certainly the grandest, with two balconies.
Attendees nearly filled the grand hall to hear the meta-learning paper by Kwonjoon Lee of University of California, San Diego; Subhransu Maji of Amazon Web Services and University of Massachusetts, Amherst; Avinash Ravichandran of Amazon Web Services; and Stefano Soatto of Amazon Web Services and University of California, Los Angeles.
“RePr: Improved Training of Convolutional Filters”
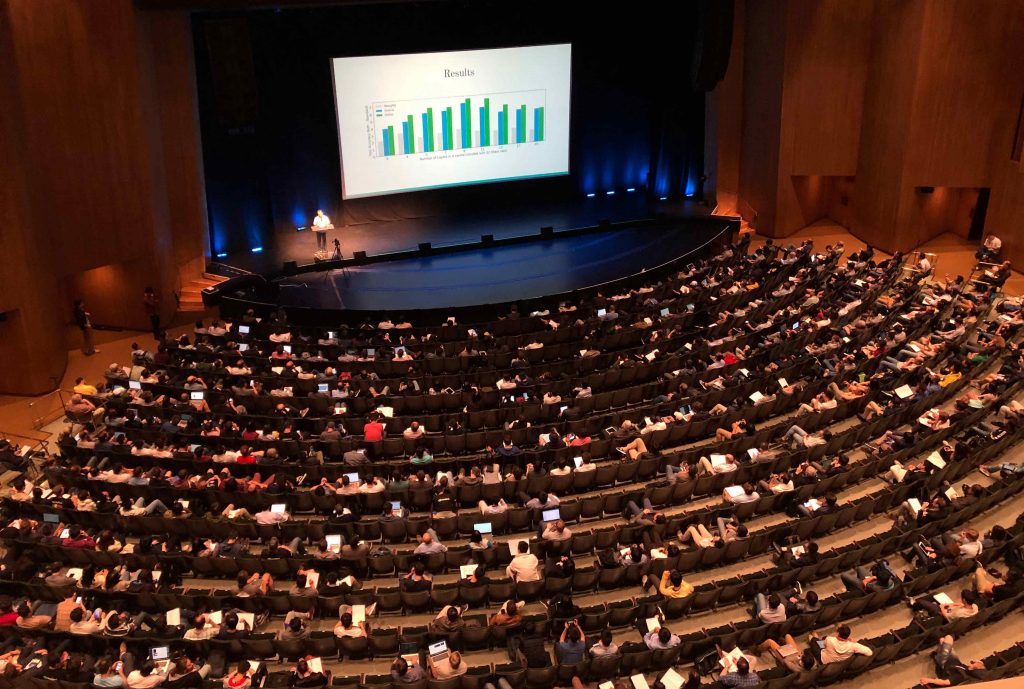
The view from the top balcony of the Terrace Theater was impressive as the oral presentation of the paper by Aaditya Prakash and James Storer, both of Brandeis University, and Dinei Florencio and Cha Zhang, both of Microsoft Research.
“A well-trained Convolutional Neural Network can easily be pruned without significant loss of performance. This is because of unnecessary overlap in the features captured by the network’s filters. Innovations in network architecture such as skip/dense connections and Inception units have mitigated this problem to some extent, but these improvements come with increased computation and memory requirements at run-time. We attempt to address this problem from another angle – not by changing the network structure but by altering the training method,” they wrote.
“Fast Spatially-Varying Indoor Lighting Estimation”
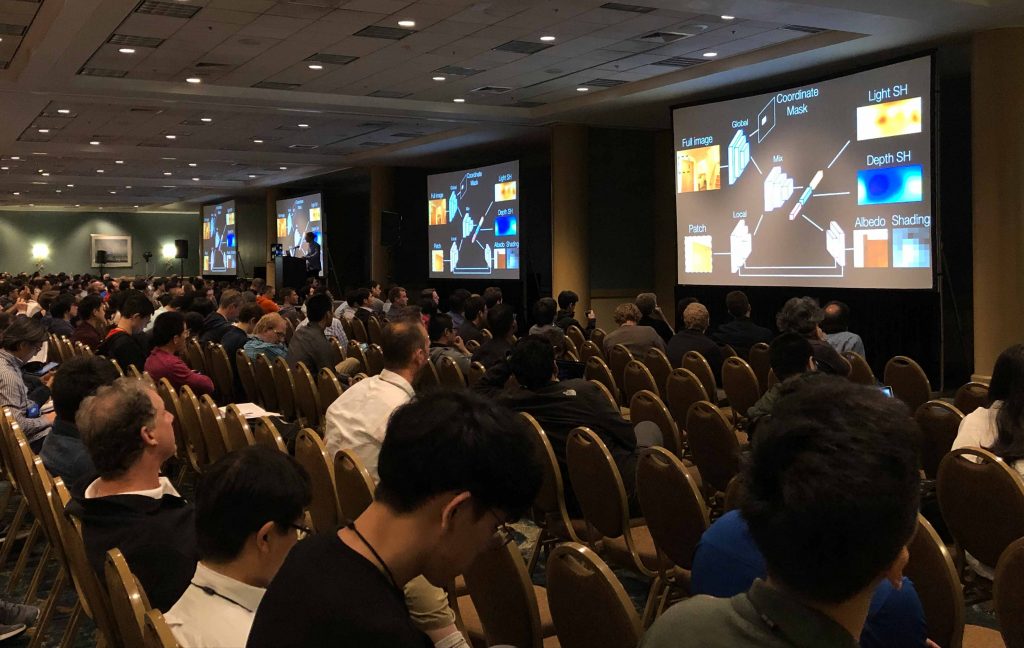
The conference’s vast size required large ballrooms to hold audiences.
Hundreds of listeners packed into the convention center’s Promenade Ballroom to hear a presentation on “Fast Spatially-Varying Indoor Lighting Estimation” by Kalyan Sunkavalli, Sunil Hadap, and Nathan Carr, all of Adobe Research; and Mathieu Garon and Jean-Francois Lalonde, both of Universite Laval.
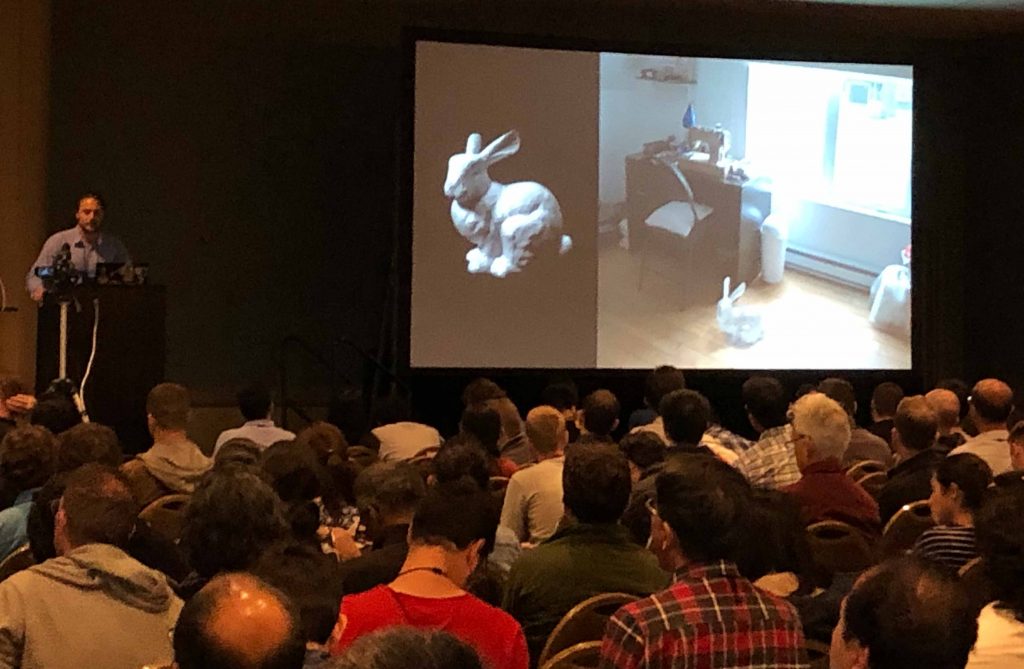
We propose a real-time method to estimate spatially varying indoor lighting from a single RGB image. Given an image and a 2D location in that image, our CNN estimates a 5th order spherical harmonic representation of the lighting at the given location in less than 20ms on a laptop mobile graphics card,” the authors said in an abstract.
“MeshAdv: Adversarial Meshes for Visual Recognition”
The ballrooms, in fact, needed at least four screens so that the researchers and others in the audience could adequately see the visually rich slide-show presentations, including “MeshAdv: Adversarial Meshes for Visual Recognition” by Chaowei Xiao, Dawei Yang, and Mingyan Liu, all of the University of Michigan, Ann Arbor; Bo Li of the University of Illinois at Urban-Champaign; and Jia Deng of Princeton University.
“Highly expressive models such as deep neural networks (DNNs) have been widely applied to various applications. However, recent studies show that DNNs are vulnerable to adversarial examples, which are carefully crafted inputs aiming to mislead the predictions,” the researchers wrote.
“High-Quality Face Capture Using Anatomical Muscles”
His pitch was catchy.
“How do you solve the problem of ‘Uncanny Valley’?” paper author Michael Bao of Stanford University and Industrial Light & Magic asked his audience in the Grand Ballroom of the convention center.
His presentation was enhanced by four giant screens, including two of which audience members could walk behind and see his presentation in reverse.
“Muscle-based systems have the potential to provide both anatomical accuracy and semantic interpretability as compared to blendshape models; however, a lack of expressivity and differentiability has limited their impact. Thus, we propose modifying a recently developed rather expressive muscle-based system in order to make it fully-differentiable,” said paper authors Bao; Matthew Cong and Stephane Grabli, both of Industrial Light & Magic; and Ronald Fedkiw of Stanford and Industrial Light & Magic.
“FML: Face Model Learning from Videos”
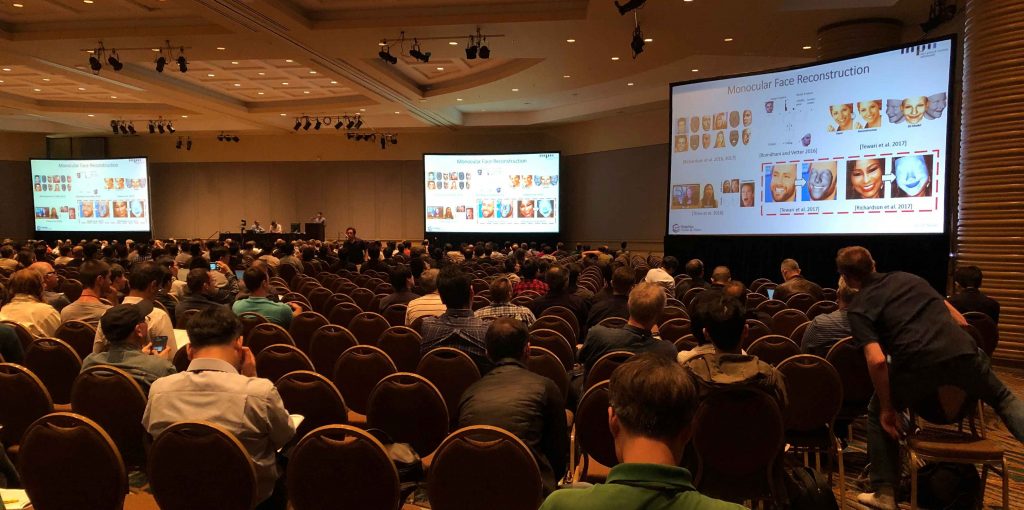
Ayush Tewari of the Max Planck Institute for Informatics, Saarland Informatics Campus, led the oral session for a paper written by him and several colleagues from Technicolor, Valeo.ai, and Stanford University.
“Monocular image-based 3D reconstruction of faces is a long-standing problem in computer vision. Since image data is a 2D projection of a 3D face, the resulting depth ambiguity makes the problem ill-posed. Most existing methods rely on data-driven priors that are built from limited 3D face scans. In contrast, we propose multi-frame video-based self-supervised training of a deep network that (i) learns a face identity model both in shape and appearance while (ii) jointly learning to reconstruct 3D faces,” wrote Tewari, Florian Bernard, Pablo Garrido, Gaurav Bharaj, Mohamed Elgharib, Hans-Peter Seidel, Patrick Perez, Michael Zollhofer, and Christian Theobalt.
Read more related articles about CVPR:
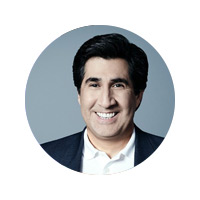
About Michael Martinez
Michael Martinez, the editor of the Computer Society’s Computer.Org website and its social media, has covered technology as well as global events while on the staff at CNN, Tribune Co. (based at the Los Angeles Times), and the Washington Post. He welcomes email feedback, and you can also follow him on LinkedIn.
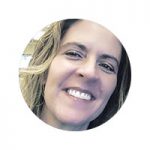
About Lori Cameron
Lori Cameron is Senior Writer for IEEE Computer Society publications and digital media platforms with over 20 years of technical writing experience. She is a part-time English professor and winner of two 2018 LA Press Club Awards. Contact her at l.cameron@computer.org. Follow her on LinkedIn.