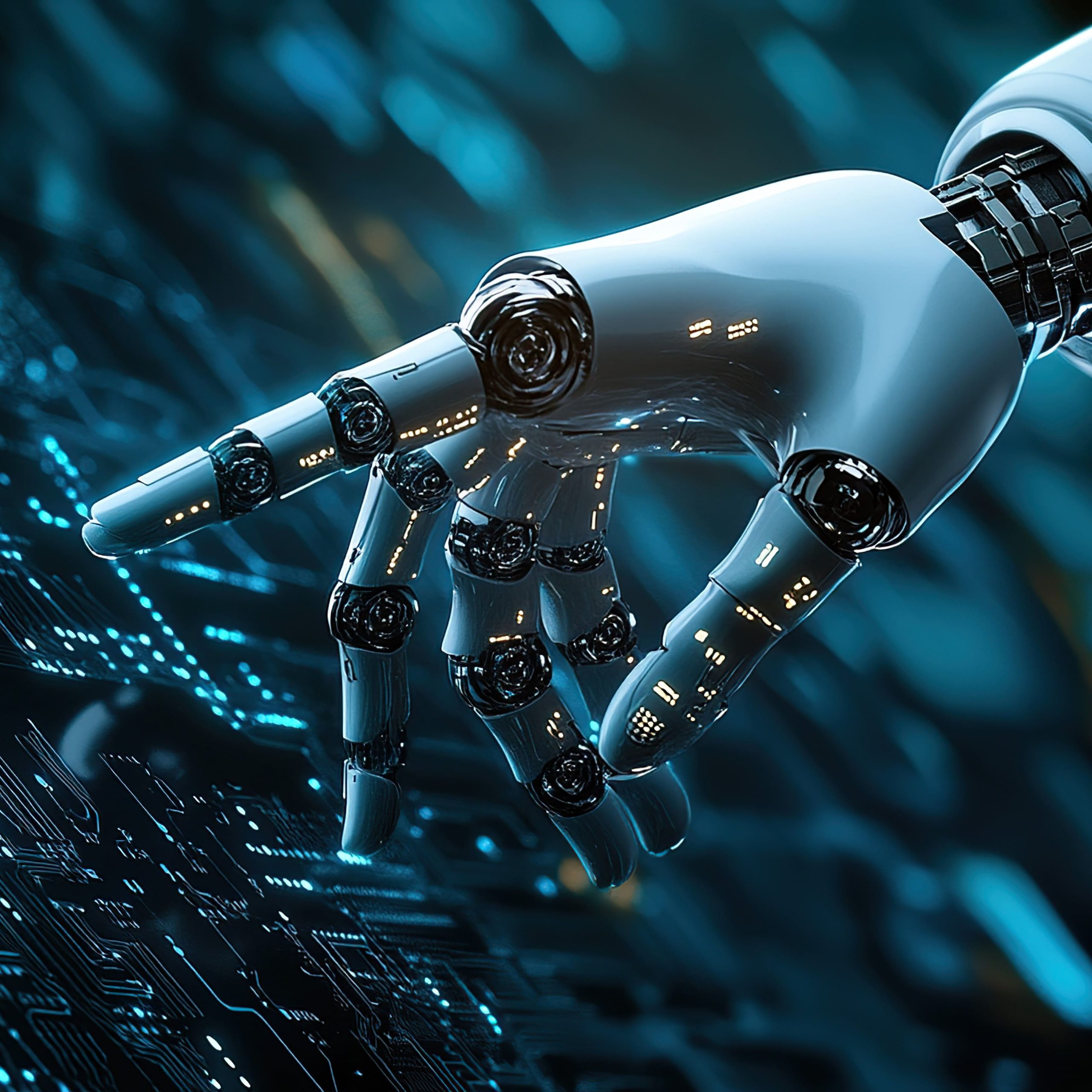
Incident management is a crucial aspect of IT operations, involving the management of incidents that can disrupt services and impact business continuity. This encompasses monitoring systems, identifying issues, analyzing root causes, implementing remediation actions, and documenting resolutions.
Effective incident management is essential for maintaining system stability, minimizing downtime, and ensuring optimal performance. However, traditional incident management approaches often struggle to keep up with the complexity and scale of modern IT environments, leading to longer resolution times.
Enter AIOps — Artificial Intelligence for IT Operations. It leverages AI and machine learning to collect and analyze vast amounts of data from various sources to identify patterns, predict issues, and automate resolutions. This enables IT teams to manage incidents more efficiently and with greater accuracy — before they escalate.
In this blog, we’ll discuss five things you must know about using AIOps for incident management.
-
Proactive Monitoring and Early Detection
AIOps platforms continuously monitor and analyze large volumes of data from various sources, such as log files, performance metrics, and event data. This allows companies to detect potential issues before they escalate into major incidents, minimize their impact, and ensure uninterrupted service delivery.
For example, an AIOps system monitoring a cloud-based e-commerce platform could detect unusual spikes in CPU utilization or abnormal response times, indicating a potential performance issue or impending system failure. This early detection would allow for proactive intervention and remediation before the issue escalates and impacts customers.
Using an AI observability platform provides more accurate and intelligent incident detection. It collects and analyzes extensive observability data from various sources, which provides a comprehensive view of the entire IT ecosystem — enabling a deeper understanding of system performance.
Further, AIOps platforms can continuously learn and adapt their incident detection models based on new observability data, incident resolutions, and feedback from IT teams. This iterative learning process enhances the accuracy and effectiveness of the AIOps platform, enabling it to make better decisions and provide more accurate recommendations.
-
Automated Remediation and Self-Healing
The best AIOps platform can go beyond simply detecting and diagnosing incidents. They can also automate remediation actions based on predefined rules, playbooks, or machine learning models. This capability enables self-healing systems that can automatically resolve multiple issues without human intervention, reducing mean time to resolution (MTTR) and minimizing operational disruptions.
For example, a global e-commerce company experiences high traffic during holiday sales. Their AIOps system is set to monitor application performance and user experience metrics. When traffic surges beyond typical levels, the system automatically scales out its web servers and adjusts database capacity in real-time to handle the increased load. Additionally, if any bugs are detected during this period, the AIOps system can trigger bug management processes to swiftly identify and rectify the issues.
This automated response ensures that customers continue to experience fast, reliable service despite the spike in demand, without requiring immediate human intervention.
To implement automated responses:
- Create detailed playbooks outlining the steps for resolving specific incidents. And, test them thoroughly to ensure they work as intended under various scenarios.
- Use machine learning models to analyze past incidents and outcomes and train them to predict effective remediation actions based on current conditions.
- Regularly review and update automated remediation rules and playbooks based on new data and incident outcomes to keep pace with evolving IT environments.
-
Predictive Analytics and Capacity Planning
Predictive analytics, the cornerstone of AIOps, leverages historical data and advanced algorithms to forecast potential future issues and capacity requirements. It enables proactive planning and resource allocation, ensuring that systems and infrastructure are adequately prepared to handle anticipated workloads or events.
This helps organizations support strategic decision-making and eliminate the risk of performance degradation by addressing potential issues before they impact users.
For example, an AIOps platform monitoring a database cluster could predict future storage requirements based on historical growth patterns and usage trends. This allows administrators to plan for capacity expansions before running out of disk space.
Here’s how you can implement it:
- Ensure your AIOps platform is integrated with all relevant data sources, including performance metrics, usage logs, and historical incident records.
- Utilize the platform’s machine learning capabilities to develop and train predictive models based on historical data.
- Continuously monitor predictions and compare them against actual outcomes. Adjust models and thresholds as necessary to improve accuracy.
-
Incident Prioritization
AIOps can intelligently prioritize and categorize incidents based on their severity, impact, and potential business consequences. It analyzes the technical details of incidents, such as error codes, system metrics, and failure rates, to determine their severity. This involves evaluating the immediate impact on system performance and functionality.
AIOps platforms also incorporate business rules and priorities to understand the potential consequences of incidents. For example, incidents affecting revenue-generating services or regulatory compliance are given higher priority.
-
Collaboration and Knowledge Sharing
Effective incident management is also about how well IT teams can collaborate and share knowledge.
AIOps platforms often integrate with collaboration tools and knowledge management systems, facilitating seamless communication and knowledge sharing among IT teams.
This reduces the time spent on manual ticket creation and ensures that incidents are addressed by the right experts.
Final Thoughts
As IT environments grow in complexity, incident management has become increasingly challenging. By understanding and leveraging the capabilities of AIOps, organizations can enhance their IT operations, reduce downtime, and deliver exceptional service levels.
So, start by integrating AIOps into your existing workflows, continuously learn and adapt, and watch as your incident management processes become more efficient and effective.
Disclaimer: The author is completely responsible for the content of this article. The opinions expressed are their own and do not represent IEEE’s position nor that of the Computer Society nor its Leadership.