Digital twins and their required software are one of the most in-demand business tools right now. According to IOT Analytics, there was a 71% increase in the standalone digital twin software market from 2020 to 2022. And it’s still growing, thanks to the versatility of what a digital twin can mean for a business.
As we’re going to look at in a moment, there are applications for multifunctional digital twins across industries. Businesses as varied as retailers, aerospace manufacturers, design consultancies, and more have taken on digital twins and are using them successfully.
Yet, a digital twin being versatile also means they can be difficult to classify and explain, which presents a challenge if you’re the one making or sourcing a digital twin for your business. So, let’s start by defining what we mean.
Defining an Accurate Digital Twin
The simplest description of a digital twin is that it’s a digital copy of a real building, space, or really any 2D or 3D object. However, we want to talk about more advanced multifunctional digital twins.
There are a few standardized ways of classifying digital twins. For example, in terms of IoT classification, digital twins are described as a “dimensional” object. Practically, that means there are three dimensions to a digital twin model. In a diagram, this would be represented by an x, y, and z axis like this.
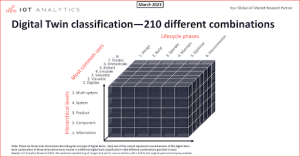
That means that when a digital twin model is built with this structure in mind, it can be built out across all three dimensions from its core components. In engineering terms, this opens up a lot of possibilities. Yet, from the diagram alone, the concept is not that easy to understand.
So, to make this a usable reality for business, it requires some further explanation. It also needs some underlying technology to make a multifunctional digital twin, so let’s look at those four areas first.
Want More Tech News? Subscribe to ComputingEdge Newsletter Today!
The Four Key Technologies For Digital Twin Use
A nice-looking copy of a product or office space can be a great illustration. However, it doesn’t do much more than that on its own.
The more advanced digital twin that you need, the more layers get added to your three-dimensional object. That means that all four of these technologies will eventually have to be a part of your design.
1. The Internet of Things (IoT)
IoT technology involves physical objects or systems being fitted with sensors, which gather data in real time. The sensors measure a wide range of parameters, such as temperature, pressure, humidity, and vibration. The data collected is then used to create a digital twin, which is able to replicate the physical object or system’s own behavior.
This often involves using deep learning to analyze and use this data. What is deep learning? It’s a machine learning technique that involves training neural networks with many layers. Keras early stopping can be used to stop training a deep learning model when it is no longer improving on a validation set, thus preventing it from overfitting to the training set.
2. Cloud Storage and Retrieval Solutions
Cloud storage and retrieval solutions allow the large amounts of data collected from IoT sensors to be stored and accessed for use in creating and managing digital twins.
Cloud storage and retrieval solutions also offer automation tips such as automating backups, automating data migrations, and automating scaling of storage resources.
3. Extended, Augmented, and Mixed Reality (XR)
AR is an exciting technology increasingly used to visualize digital twins in real-world environments. AR technologies can be used to overlay virtual images onto real-world objects, allowing users to interact with digital twins more intuitively and immersively.
For example, AR can be used to visualize digital twins of complex machinery or equipment in real-world environments. Technicians can use AR-enabled devices such as smart glasses to view a digital twin overlayed onto the physical equipment—allowing them to identify and diagnose issues more quickly and accurately.
4. Artificial Intelligence (AI): Machine Learning and Deep Learning
Artificial intelligence analyzes data collected by IoT sensors and provides insights into the behavior of physical objects or systems.
Machine learning (ML), a subfield of AI, involves training models to make predictions based on patterns in data.
ML engineers usually collaborate with various professionals, such as data engineers, data architects, data scientists, and data analysts within a team. By acting as a link between operational AI systems and data scientists, machine learning engineers enable the effective creation and delivery of trained models to end users.
Deep learning is used for complex tasks such as image recognition and image annotation. Image annotation involves labeling images with descriptive tags to provide context and enable efficient image search and retrieval.
By combining image annotation and machine learning with digital twins, industries can use annotated images to train machine learning models to identify and analyze objects and patterns within digital twins, helping to optimize maintenance schedules and predict equipment failures.
5 Digital Twin Use Cases
There are many use cases for digital twin technology, which benefits a variety of industries.
Here are some cross-industry use cases of digital twin technology:
1. Design
Digital twin technology enables you to create higher-quality products, buildings, or even entire cities. By creating a simulation of a system or a physical object, designers can test different design scenarios, identify potential design flaws, and make improvements before construction begins.
2. Operations
Used in the operations phase, digital twin tech with secure remote access can monitor the performance of assets, systems, and processes in real time. This can help companies identify and address performance issues (such as uptime, energy consumption, and maintenance requirements) before they lead to downtime or production losses.
Digital twins can also be used to optimize performance by testing different scenarios and identifying opportunities for improvement.
3. Logistics and Supply Chain
By creating a digital twin of a supply chain network, companies can simulate and test different scenarios to identify bottlenecks and inefficiencies and optimize the flow of goods and materials in supply chains.
A business might leverage digital twinning to simulate the impact of a disruption in the supply chain, such as a delay in the delivery of raw materials or a breakdown in the transportation network. The digital twin can be used to evaluate the impact of the disruption on the entire supply chain network and identify alternative routes and suppliers to mitigate the impact of the disruption.
4. Service
By creating a digital twin of a service process, companies can identify opportunities to improve and optimize the delivery of services.
For example, in Israel, digital twin technology has grown in popularity over the past few years, with many companies and organizations adopting it to improve their operations and services. Many companies with an .il domain in Israel have used digital twin technology to optimize their manufacturing processes, improve their supply chain operations, and enhance the customer experience.
5. Leisure
Digital twin technology can also be used in the leisure industry to create immersive experiences for customers. For example, amusement parks can use digital twin technology to simulate rides and attractions, allowing customers to experience them virtually before they visit the park.
This allows customers to plan their visit and prioritize the attractions they want to experience, which can lead to a more satisfying experience.
Real-World Digital Twin Examples
Example 1: Utilities: Energy Output Optimization E.ON
E.ON implemented digital twinning to monitor the health of its assets and collect performance data from 110 kV power transformers.This allowed the firm to switch from a reactive, time-based method of asset management to an approach based on prevention, prediction, and risk.
Example 2: Medicine: Deep Learning Image Analysis KeAi
Technology to improve healthcare is nothing new. However, in this innovative use case, scientists developed a smart healthcare system based on digital twin technology, which could integrate with medical devices. The aim was to gather data about the patient’s health, and the configuration and upkeep of the device/machine/system. It allowed X-rays to be further analyzed by a deep learning model to detect the infection of COVID-19.
Example 3: Civil Engineering: Italy’s Smart Trains Ferrovie Dello Stato
A nationwide railway operator decided to create a digital twin of its railway infrastructure, which included some 16,000 km of track. The digital twin takes into account all infrastructure—from stations, bridges, and tunnels to signals, switches, IT systems, and electrification hardware.
The digital replica now enables a reliable stream of accurate geographic data throughout the rail network. Giving technicians and managers the tools that help them achieve work sooner – driving efficiency and nurturing the railway’s financial health.
Digital Twins: An Exciting Technology
Digital twins are becoming increasingly popular across various industries.
This is due to their ability to simulate the behavior of physical objects and systems. They allow companies to optimize performance and reduce downtime. From monitoring the performance of renewable energy assets in the energy industry to predicting equipment failures in the manufacturing industry, digital twins have a wide range of use cases.
With the integration of technologies such as machine learning and image annotation, digital twins are becoming even more powerful. These tech-driven insights can help companies make data-led decisions and stay ahead of the competition. The future of digital twins looks promising, with the potential to revolutionize the way we design, build, and maintain physical assets and systems.
Disclaimer: The author is completely responsible for the content of this article. The opinions expressed are their own and do not represent IEEE’s position nor that of the Computer Society nor its Leadership.