Experts believe that Artificial Intelligence (AI) and Machine Learning (ML) have both negative and positive effects on cybersecurity. AI algorithms use training data to learn how to respond to different situations. They learn by copying and adding additional information as they go along. This article reviews the positive and the negative impacts of AI on cybersecurity.
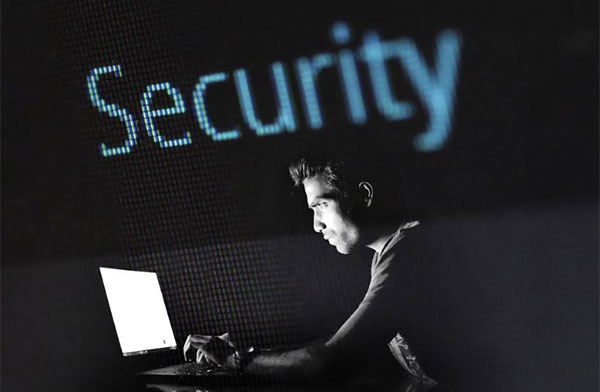
Image Source
Main Challenges Cybersecurity Faces Today
Attacks are becoming more and more dangerous despite the advancements in cybersecurity. The main challenges of cybersecurity include:
- Geographically-distant IT systems—geographical distance makes manual tracking of incidents more difficult. Cybersecurity experts need to overcome differences in infrastructure to successfully monitor incidents across regions.
- Manual threat hunting—can be expensive and time-consuming, resulting in more unnoticed attacks.
- Reactive nature of cybersecurity—companies can resolve problems only after they have already happened. Predicting threats before they occur is a great challenge for security experts.
- Hackers often hide and change their IP addresses—hackers use different programs like Virtual Private Networks (VPN), Proxy servers, Tor browsers, and more. These programs help hackers stay anonymous and undetected.
AI and Cybersecurity
Cybersecurity is one of the multiple uses of artificial intelligence. A report by Norton showed that the global cost of typical data breach recovery is $3.86 million. The report also indicates that companies need 196 days on average to recover from any data breach. For this reason, organizations should invest more in AI to avoid waste of time and financial losses and.
AI, machine learning, and threat intelligence can recognize patterns in data to enable security systems learn from past experience. In addition, AI and machine learning enable companies to reduce incident response times and comply with security best practices.
How AI Improves Cybersecurity
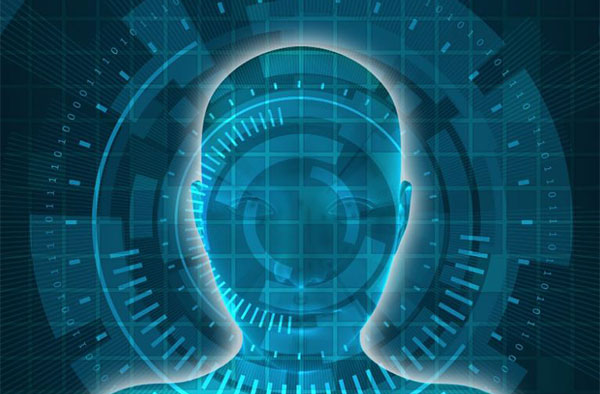
Image Source
Threat hunting
Traditional security techniques use signatures or indicators of compromise to identify threats. This technique might work well for previously encountered threats, but they are not effective for threats that have not been discovered yet.
Signature-based techniques can detect about 90% of threats. Replacing traditional techniques with AI can increase the detection rates up to 95%, but you will get an explosion of false positives. The best solution would be to combine both traditional methods and AI. This can result in 100% detection rate and minimize false positives.
Companies can also use AI to enhance the threat hunting process by integrating behavioral analysis. For example, you can leverage AI models to develop profiles of every application within an organization’s network by processing high volumes of endpoint data.
Want more tech news? Subscribe to ComputingEdge Newsletter Today!
Vulnerability management
20,362 new vulnerabilities were reported in 2019, up 17.8% compared to 2018. Organizations are struggling to prioritize and manage the large amount of new vulnerabilities they encounter on a daily basis. Traditional vulnerability management methods tend to wait for hackers to exploit high-risk vulnerabilities before neutralizing them.
While traditional vulnerability databases are critical to manage and contain known vulnerabilities, AI and machine learning techniques like User and Event Behavioral Analytics (UEBA) can analyze baseline behavior of user accounts, endpoint and servers, and identify anomalous behavior that might signal a zero-day unknown attack. This can help protect organizations even before vulnerabilities are officially reported and patched.
Data centers
AI can optimize and monitor many essential data center processes like backup power, cooling filters, power consumption, internal temperatures, and bandwidth usage. The calculative powers and continuous monitoring capabilities of AI provide insights into what values would improve the effectiveness and security of hardware and infrastructure.
In addition, AI can reduce the cost of hardware maintenance by alerting on when you have to fix the equipment. These alerts enable you to repair your equipment before it breaks in a more severe manner. In fact, Google reported a 40 percent reduction in cooling costs at their facility and a 15 percent reduction in power consumption after implementing AI technology within data centers in 2016
Network security
Traditional network security has two time-intensive aspects, creating security policies and understanding the network topography of an organization.
- Policies—security policies identify which network connections are legitimate and which you should further inspect for malicious behavior. You can use these policies to effectively enforce a zero-trust model. The real challenge lies in creating and maintaining the policies given the large amount of networks.
- Topography—most organizations don’t have the exact naming conventions for applications and workloads. As a result, security teams have to spend a lot of time determining what set of workloads belong to a given application.
Companies can leverage AI to improve network security by learning network traffic patterns and recommending both functional grouping of workloads and security policy.
Drawbacks and Limitations of Using AI for Cybersecurity
There are also some limitations that prevent AI from becoming a mainstream security tool:
- Resources—companies need to invest a lot of time and money in resources like computing power, memory, and data to build and maintain AI systems.
- Data sets—AI models are trained with learning data sets. Security teams need to get their hands on many different data sets of malicious codes, malware codes, and anomalies. Some companies just don’t have the resources and time to obtain all of these accurate data sets.
- Hackers also use AI—attackers test and improve their malware to make it resistant to AI-based security tools. Hackers learn from existing AI tools to develop more advanced attacks and attack traditional security systems or even AI-boosted systems.
- Neural fuzzing—fuzzing is the process of testing large amounts of random input data within software to identify its vulnerabilities. Neural fuzzing leverages AI to quickly test large amounts of random inputs. However, fuzzing has also a constructive side. Hackers can learn about the weaknesses of a target system by gathering information with the power of neural networks. Microsoft developed a method to apply this approach to improve their software, resulting in more secure code that is harder to exploit.
Conclusion
Artificial intelligence and machine learning can improve security, while at the same time making it easier for cybercriminals to penetrate systems with no human intervention. This can bring significant damage to any company. Getting some kind of protection against cyber criminals is highly recommended if you want to reduce losses and stay in business.
Author Bio
Eddie Segal is an electronics engineer with a Master’s Degree from Be’er Sheva University, a big data and web analytics specialist, and also a technology writer. In my writing I cover subjects ranging from cloud computing to agile development to cybersecurity and deep learning.
More Related Articles